In our cloud reachability study, we performed extensive global client-to-cloud latency measurements towards 189 datacenters from nine major cloud providers using 8,000+ RIPE Atlas probes. Our goal was to evaluate the suitability of modern cloud environments for latency-sensitive applications. Here, we share the most interesting results of the study.
We conducted a large-scale measurement study over the span of 12 months, analysing the reachability of nine major cloud providers, spanning 189 datacenters deployed in 28 countries. We used more than 8,000 RIPE Atlas probes deployed in 184 countries to periodically measure user-to-cloud latency (ping) and path length (traceroute) over ICMP and TCP. Our collected dataset reaches almost 60 GB in size and is publicly available here. A collection of related works to this article can be found at this website.
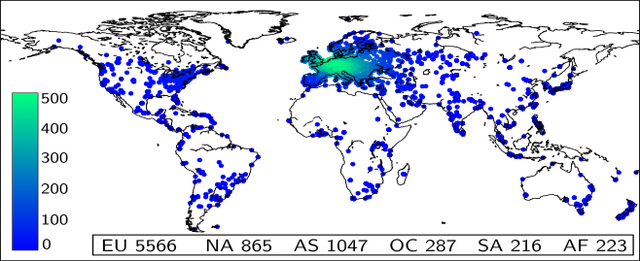
Latency-Sensitive Applications in the Cloud
One of the aims of the study was to look at the spread of cloud networks and identify their suitability for the deployment of latency-critical applications. To do this, we compared the obtained latency distributions against three well-known timing thresholds: namely, human reaction time (HRT) (∼250 ms); human perceivable latency (HPL) (∼100 ms); and motion-to-photon (MTP) (∼20 ms). Additional information on latency-sensitive applications can be found in our HotNets 2020 paper.
Figure 2 (below) illustrates the global RTT distribution from all probes in our dataset, one for each timing threshold. Different colour groups denote different percentiles of the distribution. The results suggest that almost every country across the globe can consistently reach the closest cloud datacenter within the boundaries of the HRT. In fact, only two (out of 184) countries in our dataset achieve the HRT less than 25% of the time, and three countries lie between 50 and 75%.
For HPL, we observe that the cloud maturity level changes slightly compared to the HRT. The distribution of RTTs degrades only for certain countries, mainly clustered in Central Africa, the Middle East, and South America. Specifically, 140 countries achieve RTTs consistently within the boundaries of the HPL, six achieve that only 50 to 75% of the time, another six countries only 25 to 50% and, 16 countries fail to reliably meet the HPL threshold.
The distribution changes substantially for the MTP threshold, where only 24 countries can consistently meet the timing deadline (75–100%). Conversely, 125 countries fail to meet the threshold (0–25% of the sample), while the remaining 25 countries can reach cloud within MTP between 25–75% of the times.
Takeaway — The current cloud infrastructure is able to deliver network latency compliant with both HRT and HPL safely. However, only a small minority of countries reliably meet the MTP threshold, suggesting that either cloud deployment or network should be improved.

Private Backbone vs. Public Internet
We also addressed the impact of network backbone infrastructure on latency performance. Many cloud providers deploy extensive private wide area network (WAN) to interconnect their datacenters providing clients fast-track paths to services hosted in their infrastructure. Table 1 lists the network backbone type used by the different cloud providers targeted in our measurements.
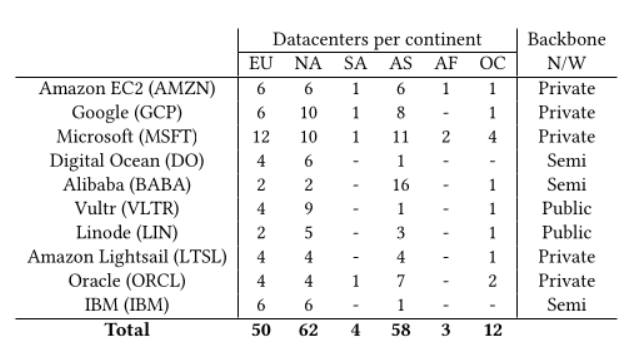
In Figure 3, we show the distribution of the latencies achieved by RIPE Atlas probes, at continental granularity, towards the closest datacenter of every cloud provider. Since the aim of this work is not to provide a benchmark study comparing the performance of different cloud operators across the globe, we do not probe all cloud regions in this analysis. Instead, we only draw results from those regions which were found closest (in latency) to our vantage points.
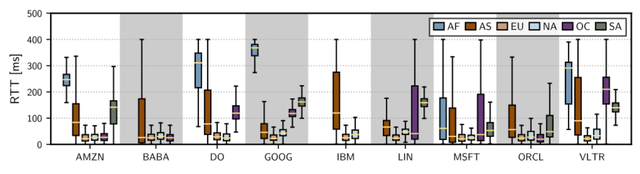
From the figure, it is evident that the availability of private network backbone in continents with extensive network deployment, like North America and Europe, does not seem to have much impact on latency performance. In fact, we find that all cloud providers exhibit similar latency distributions in these regions – accentuated more towards providers relying on the public Internet.
In Oceania, Amazon EC2, Alibaba Cloud, and Oracle achieve the least latency results while Microsoft Azure and Linode perform similarly but with higher variance. We justify their superior performance to their extensive deployment in the continent. Within Asia, almost all providers perform similarly, and we do not observe any significant benefits favouring providers with private WAN and those relying on public Internet. For South America, we probed Amazon EC2, Microsoft, and Oracle since only those have datacenters deployed within the continent. For the rest of the providers, we show latencies from South American probes to their datacenters in North America. We observe that cloud providers with local datacenter deployment perform significantly better than those with infrastructure in the neighbouring continent. A similar trend can also be observed in Africa, where providers with in-land datacenter deployment (specifically Microsoft and Amazon EC2) show much lower latency than their counterparts, which host datacenters in the neighbouring continent of Europe. It is to be noted that we draw our inferences from small-sized ICMP and TCP packets, and the impact of private backbone will be far more significant for elephant flows within the cloud infrastructure.
Takeaway — The impact of private WAN availability on cloud reachability is not as significant as otherwise assumed. In continents with dense network deployment, public Internet delivers almost similar performance compared to a cloud provider that deploys its own private network backbones. Moreover, the availability of datacenters within a continent impacts connectivity far more than the type of interconnecting network infrastructure
Cloud Access Case Studies
The United States of America
We find the US as a good object of study since it covers a large geographical area, has a large population, and has remained the focal point for major cloud providers – as reflected by the dense cloud presence within the country (Table 1). We selected the most populated regions in the US using the US Primary Statistical Areas (PSA). The federal government has defined hundreds of PSAs, which collectively house more than 80% of the total US population. We further selected 93 PSAs and collected up to 25 probes within a radius of 125 km from the centre of PSA location.
Overall, we selected 701 probes, each performing multiple ping measurements towards 15 datacenters belonging to all cloud providers within the US. Figure 4 shows the results. We show the minimum, median, and 95𝑡ℎ percentile of latency observed in every PSA. The distribution is weighted according to the population of each PSA; visually, this translates to a higher vertical step in the CDF, for a larger population. The median distribution shows that almost the entire US population has median access latency below 75 ms – well within the human-perceivable latency threshold. The differences, however, show up for the 95𝑡ℎ percentile distribution of PSAs latency as it includes probes installed in imperfect network conditions. Even in this case, ∼ 60% of the US population can reach the cloud within the coveted 100 ms threshold.
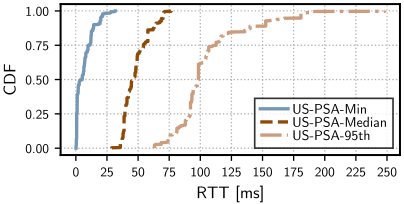
Asia
We contrast our analysis above by focusing on the state of cloud access in Asia. We carefully select seven Asian countries based on their landmass and physical distance to the cloud. Specifically, we investigate five countries with local datacenter deployments: i.e., China (48 probes); India (108 probes); Singapore (80 probes); Korea (20 probes); Japan (188 probes); Pakistan (12 probes), which directly shares borders with the country with datacenter (India); and Iran (120 probes), which is farthest from any datacenter in the continent and nearest deployment in UAE and India.
Figure 5 shows the results. It is evident from the figure that countries with locally deployed datacenters can consistently meet the HPL threshold (100 ms). On the other hand, the impact of large geographical distance from the nearest datacenter, becomes evident in countries with no in-land datacenter. For instance, only 40% of samples from Pakistan are below 100 ms, while the rest can only satisfy the HRT threshold (250 ms). Finally, Iran being the geographically farthest from any datacenter, the minimum latency to reach the cloud is ∼200 ms, and almost 30% of samples did not satisfy the HRT threshold.
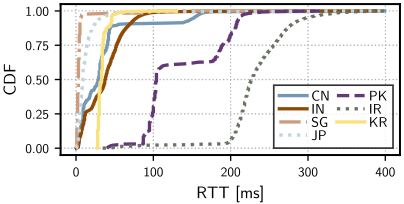
Takeaway — The current cloud presence in the US can easily support the bulk of emerging applications, bounded by HPL constraints, for the majority of the population. On the other hand, while cloud reachability in Asia is generally good for countries with local deployments (e.g., China, Korea, Japan, India), it significantly degrades with increasing geographical distance from the physical location of datacenters. Furthermore, the state of the user’s network connectivity does not seem to have much effect on cloud reachability, as evident from consistently high latencies achieved by the majority of probes of Iran.
Conclusion
We conducted a large-scale cloud reachability study with the aim to evaluate the current state of cloud connectivity globally. In our study, we targeted 189 compute-capable cloud regions of nine major cloud providers from more than 8000 globally distributed RIPE Atlas probes for a period of 12 months. Through our extensive analysis of network latency, we found that the majority of the world population can access a cloud facility within 100ms – which is a critical threshold for many future-forward latency-sensitive networked applications. Furthermore, we found that end-to-end network latency is rarely impacted by underlying network infrastructure as even providers relying on public Internet achieve similar latencies, albeit with higher variability. Our case study analysis showcased the impact of geographical distance to cloud by analysing regions with contrasting datatcenter deployment density – the USA and Asia. Our results revealed that extensive datacenter deployment is key to make cloud access latencies consistently compatible with requirements of next-generation applications, especially for Asia, South America, and Africa.
Additional Results and Artefacts
This is a short excerpt from the full research paper that was presented at The Web Conference 2021, and can be downloaded here. We invite interested readers to check the full paper since it include more latency analysis as well as the study of network paths length and the pervasiveness of the cloud networks. It is also possible to watch the video presentation broadcasted at The Web Conference at this link.
We make the collected dataset publicly available here, as well as the code for replicating our results on GitHub.
Related works to this article can be found at this website.
Comments 0
The comments section is closed for articles published more than a year ago. If you'd like to inform us of any issues, please contact us.